There is no single formula for measuring data quality, as it can be affected by a variety of factors. However, there are a few general steps that can be taken to assess the data against the requirements and determine its quality. Continue reading to learn more about these important data quality measures and how they can best be implemented for the requirements of your business or organization.
Understand the data
Data quality is a measure of how accurate and reliable your data is. Data accuracy is the degree to which the data represents reality. Data reliability is the degree to which the data is consistent and dependable.
To measure data quality, you need to understand the data. You need to understand the source of the data, the format of the data, the meaning of the data, and the use of the data. You also need to understand the quality of the data.
One common measure is data completeness. This looks at how much of the data is actually included in the set and how much is missing. Another measure is accuracy, which looks at how close the data is to the actual value. This can be done by comparing the data to a reference set or by using statistical measures. Another common measure is data timeliness. This looks at how recent the data is and how up-to-date it is. This is often important for businesses that need to make timely decisions. Finally, data volatility looks at how much the data changes over time. This is important for businesses that need to make predictions based on past trends.
Define the data
In order to measure the data quality, it is necessary to define the data quality requirements. The requirements may include accuracy, completeness, timeliness, and integrity. The data requirements should be based on the needs of the business.
Once the requirements are defined, the data can be measured against those requirements. The accuracy of the data can be measured by comparing the data against a known source. The completeness of the data can be measured by checking to see if all the required fields are populated. The timeliness of the data can be measured by comparing the data against a known time frame. The integrity of the data can be measured by checking for inconsistencies.
The data quality can be improved by addressing any deficiencies that are identified. The data quality should be monitored on an ongoing basis to ensure that it meets the requirements of the business.
Understand the business requirements.
The best way to determine what metrics are important to your organization is to consult with the business stakeholders. They will be able to tell you what is important to them and what they need from the data.
Once you have a good understanding of the business requirements, you can start to measure the data quality against those requirements. This can be done through manual reviews or automated processes. Automated processes are often more efficient and accurate, but they can be more expensive to set up and maintain.
No matter which method you use, it is important to track the results so that you can see how the data quality is improving (or deteriorating). This will help you to focus your efforts on the areas that need the most attention.
By understanding the business requirements and using the appropriate data quality metrics, you can ensure that your data is of the highest quality possible.
Define what data quality means to your organization.
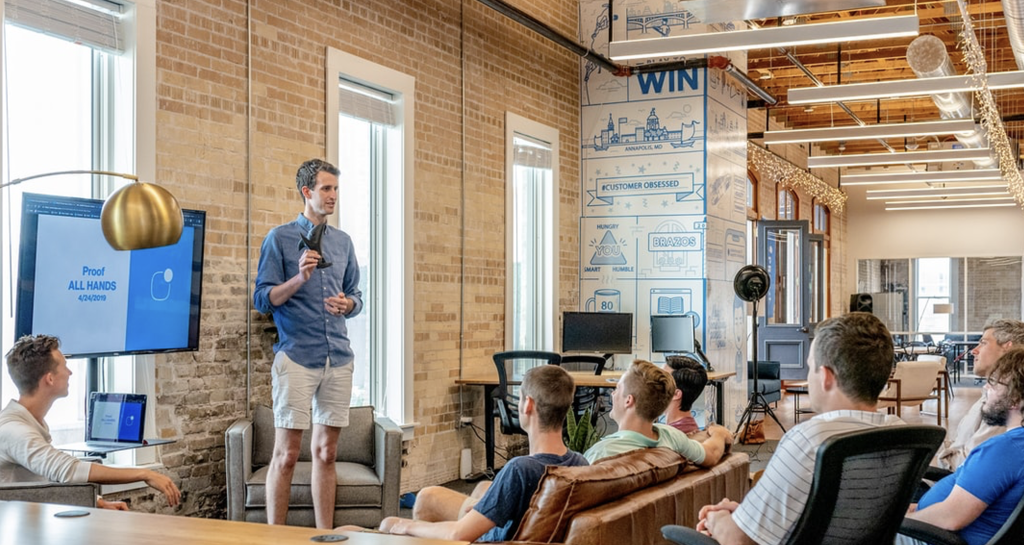
The definition of data quality depends on the organization. Some organizations may focus on accuracy, while others may focus on completeness. Some organizations may focus on the timeliness of the data, while others may focus on the usability of the data. It is important for organizations to define what data quality means to them so that they can measure it accurately.
By understanding these key areas, it is possible to measure the data quality and take steps to improve it. With accurate, consistent data, businesses can make better decisions and achieve their goals.